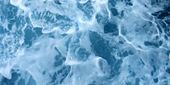
Our statistical modelling consulting services can encompass the full spectrum of problem identification, formulation, software development, modelling and appraisal, or a subset of these as appropriate. Software solutions that are developed can either be an application based on existing modelling tools and statistical programming libraries (e.g. R and Python), our in-house statistical modelling package QuinStats, or bespoke custom software.
Quintessa's general approach to statistical modelling is to work with the client to postulate a number of candidate models for the behaviour of the system in question, the precise form of which will often be motivated by physical understanding of the system. It is important that the models capture the known variability and uncertainty in the system in question. The available historical data are used to determine which of these models are consistent with the observations. It is generally better to work with several models that are consistent with the data, but which may extrapolate differently, rather than to concentrate on a single ‘best’ model. This enables the assessment of model uncertainty as well as parameter uncertainty. The complexity of the models needs to be compatible with the information in the data. When the data available are limited, it may be most suitable to use quite simple models for system evolution, but as more data become available it is often possible to justify more complex models.
An example application of Quintessa's statistical modelling and software development capabilities is CoreStats. This software is used to model the evolution of the graphite core of Advanced Gas-cooled Reactors (AGRs). Blind predictions are made ahead of reactor inspections, where the data are very expensive to obtain but cover only part of the system. The predictions are then compared with data obtained from the inspection, to enable a judgement to be made on both the performance of the models (and therefore their predictive power) and on the consistency of the new data with the historic data and current physical understanding.