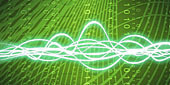
Data science is the extraction of knowledge from data, and analytics is the discovery and communication of meaningful patterns in data. Data analysis techniques are many and varied and the choice of technique for a given activity is not always obvious. Where the data are ‘unusual’ or where highly domain-specific answers are sought, often new or tailored techniques must be developed.
The term data analysis has been in use since before the advent of the computer era. Originally considered as an extension of mathematical statistics, cluster analysis and other multivariate techniques have been developed since the early 20th century. Nowadays, data analysis is generally used to describe activities in which either:
- data are used to fit and/or test mathematical models, with a view to then using the models to make predictions; or
- data are ‘mined’ to enhance and augment knowledge of the domain from which the data originate.
The first of these activities is usually approached using classical statistics, where typically a problem is proposed first, with the investigators then utilising the data to progress towards a viable model. In the latter case, the data often come first, with the problem being to try and infer structure or patterns in the data in order to make sense of the data. However, these two approaches are not exclusive, and a combination or hybrid approach can often be optimal.
As with other areas of Quintessa’s work, the key is working in partnership with our clients to develop a thorough understanding of the data and the problem to be addressed. Only with this detailed understanding can tailored techniques be developed that deliver maximal value.
A major current trend is big data analytics, aimed at gaining useful knowledge from vast quantities of digital data, where machine learning methods can be particularly valuable. While Quintessa’s mathematically based approach can be applied to such problems, our main focus to date has been on extracting the maximum value out of relatively small data sets, for which the acquisition of each data point often requires considerable time and/or resources.
For example, Quintessa has worked with EDF Energy to develop statistical models of the evolution of the graphite core of Advanced Gas-cooled Reactors (AGRs) based on measurements of the core and reactor operational data; these models continue to be calibrated to reactor data to refine their predictions, for which Quintessa provides ongoing consultancy services.